The first project in 2020 investigated the possible use of Machine Learning/Neural Networks in safety-critical avionics, looking in particular at the applicability of existing guidance such as ‘Guidelines for Development of Civil Aircraft and Systems’ ED-79A/ARP4754A and ‘Software Considerations in Airborne Systems and Equipment Certification’ ED-12C/DO-178C.
An essential finding of the previous EASA/Daedalean joint 140-page report (public version) was the identification of a W-shaped development process adapting the classical V-shaped cycle to machine learning applications:
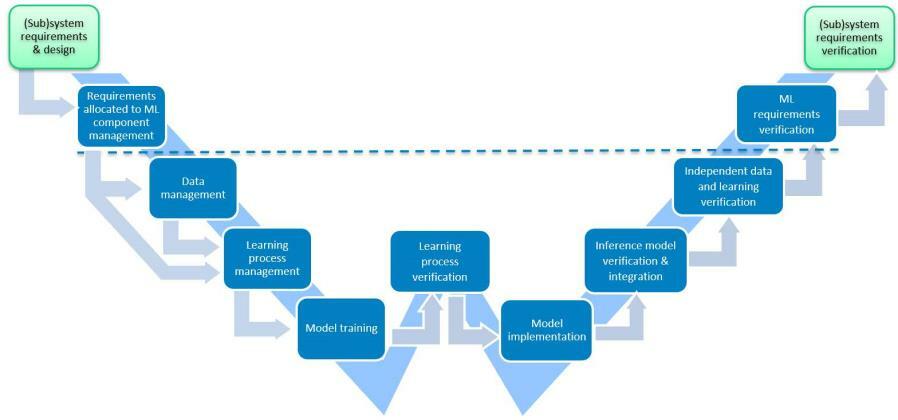
W-shaped development cycle for Learning assurance
Where the first CoDANN project showed that using neural networks in safety-critical applications is feasible, CoDANN II answers the remaining questions, reaching a conclusion on each of the following topics:
- Implementation and inference parts of the W-shaped process (hardware, software and system aspects), encompassing development and deployment aspects;
- Definition and role of explainability;
- Details on the system safety assessment process, concluding discussions on the integrating neural networks into complex systems and their evaluation in safety assessments.
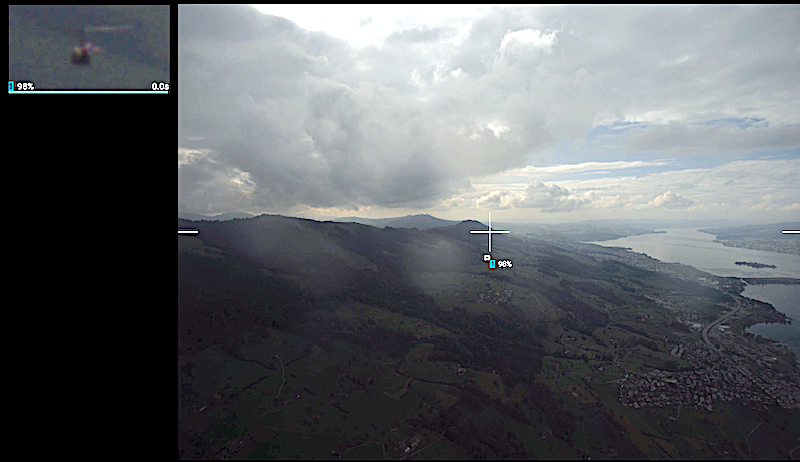
Development view of Daedalean’s visual traffic detection system: a helicopter spotted in difficult weather conditions
The visual traffic detection system developed by Daedalean served as a concrete use case. Like the visual landing guidance used as an example in the first project, this complex ML-based system is representative of future AI/ML products and illustrates the safety benefit such functions may bring to future airborne applications. Points of interest for future research activities, standards development and certification exercises have been identified.
EASA already used findings from both projects in drafting the first usable guidance for Level 1 machine learning applications released for public consultation in April 2021.
“Working with the EASA AI Task Force was again a productive exercise,” said Luuk van Dijk, CEO and founder of Daedalean. “We each contributed our expertise on aviation, safety, robotics and machine learning to arrive at a result we could not have achieved without each other. The result was a 136-page report, the major part of which has been published for the benefit of the public discussion in this field”.