The emerging advanced air mobility market is driving the convergence of airborne electronic systems that are highly integrated with unprecedented functionality. Legacy avionics systems simply can’t meet these new requirements: namely because they lack computing power. Furthermore, the transition to fully-automated and autonomous operations necessitates machine learning, enabling capabilities that traditionally are limited to human pilots, such as passively detecting and avoiding airborne traffic and identifying safe landing locations.
Daedalean leverages the power of AI to provide superhuman situational awareness systems to aerospace. In doing so, we deliver situational intelligence. Situational intelligence not only provides the ability to understand and make sense of the current environment and situation but also anticipate and react to a future situation (including a future problem). It encompasses situational awareness as well as the knowledge of how to respond. Furthermore, situational intelligence is a necessary component for achieving high automation and [intelligent] autonomy.
What does situational intelligence look like in practice? Here’s an example: imagine the aircraft suffers an engine failure. Today, the Crew Alerting System would provide a warning that an engine has failed. The pilot then takes over and makes a number of decisions: is an emergency landing required? Can we make it to a nearby airport? Where can we land safely? The pilot must make these decisions quickly and in a stressful situation. However, the future AI-enhanced situationally-intelligent system would do more than alert the pilot; it would provide assistance and guidance: an engine warning alert would immediately prompt recommendations on whether to continue to the nearest airport or initiate an emergency landing, at which point the landing site finder would provide recommendations for safe landing locations. Once the pilot confirmed a target location, the landing system would provide guidance to a safe landing.
Daedalean leverages the power of AI to provide superhuman situational awareness systems to aerospace. In doing so, we deliver situational intelligence. Situational intelligence not only provides the ability to understand and make sense of the current environment and situation but also anticipate and react to a future situation (including a future problem). It encompasses situational awareness as well as the knowledge of how to respond. Furthermore, situational intelligence is a necessary component for achieving high automation and [intelligent] autonomy.
What does situational intelligence look like in practice? Here’s an example: imagine the aircraft suffers an engine failure. Today, the Crew Alerting System would provide a warning that an engine has failed. The pilot then takes over and makes a number of decisions: is an emergency landing required? Can we make it to a nearby airport? Where can we land safely? The pilot must make these decisions quickly and in a stressful situation. However, the future AI-enhanced situationally-intelligent system would do more than alert the pilot; it would provide assistance and guidance: an engine warning alert would immediately prompt recommendations on whether to continue to the nearest airport or initiate an emergency landing, at which point the landing site finder would provide recommendations for safe landing locations. Once the pilot confirmed a target location, the landing system would provide guidance to a safe landing.
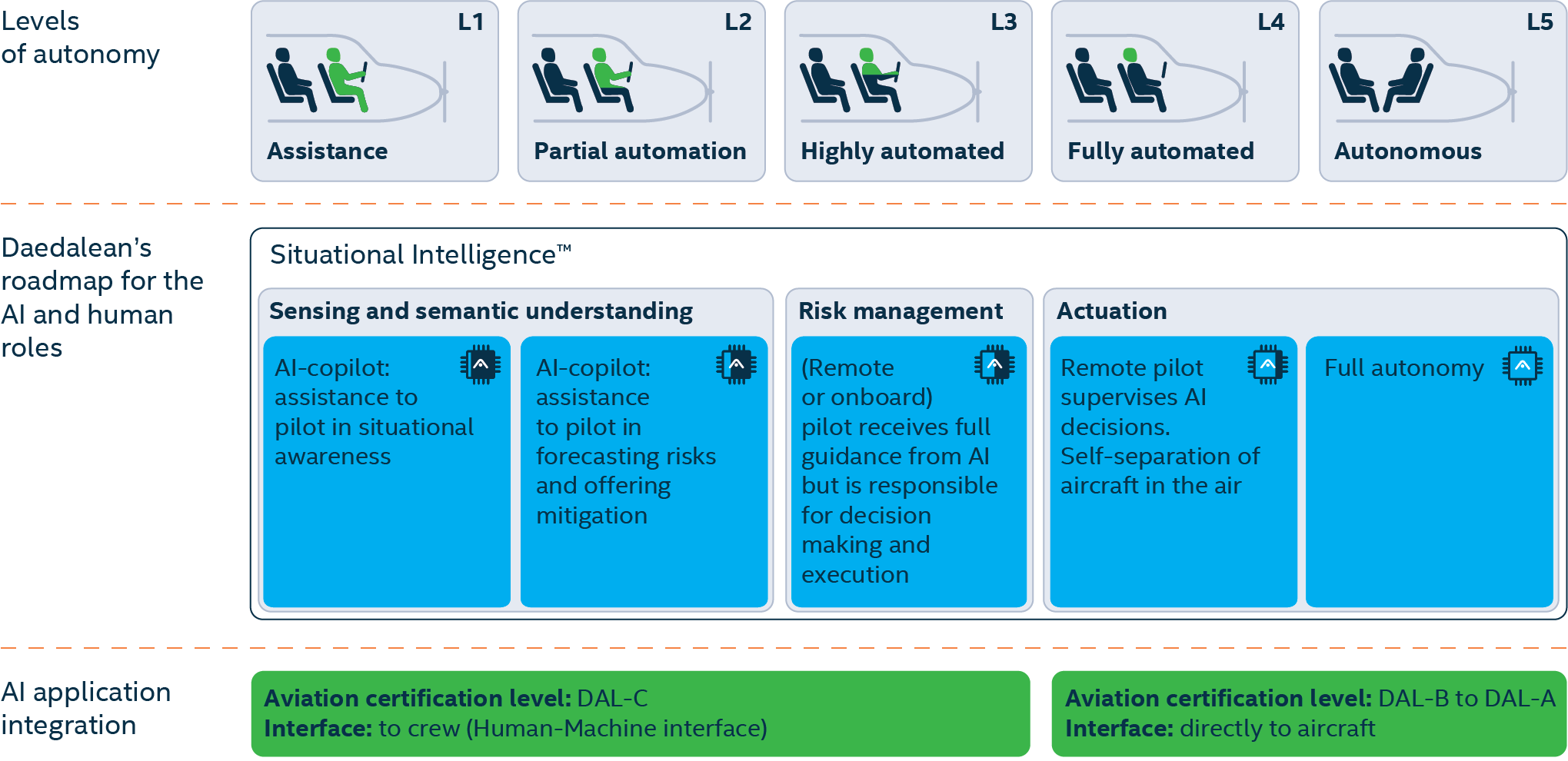
And here’s the best part: situationally-intelligent systems such as the above would operate with either human or machine pilots and at all levels of automation toward autonomy. This isn’t some pipe dream we’ll realize in the distant future. Daedalean is delivering situational intelligence today.
As we bring certifiable situational intelligence to aerospace, we are closing the gap between artificial intelligence, namely machine learning, and embedded aerospace computing. We’ve learned quite a bit along this journey.
Embedded computing professionals still need to gain experience with artificial intelligence (AI). They need to gain knowledge on this topic and may need help building systems that support and incorporate AI.
After years of working with the algorithms for the application layer and testing them on various hardware in the lab and onboard flying aircraft, Daedalean came to understand how the application capabilities can be enabled from a silicon perspective. Based on our use cases for machine learning, particularly these vision-based situational intelligence systems, we can specify their hardware requirements and offer the reference architecture – a problem statement that this market had yet to solve.
Today, Intel® published the whitepaper written in collaboration with Daedalean. We provide real-world examples of how our AI-powered situational awareness technology can be used in certifiable applications, such as vision-based traffic detection, navigation, and landing guidance included in our Situational Intelligence™ suite. Based on these use cases, we outline the specific hardware requirements sufficient to support the named capabilities.
Aircraft embedded developers, including avionics OEMs, COTS solutions providers, and airframers/systems integrators, developing computing systems face specific challenges related to airworthiness – their equipment needs to be small in size and weight, physically robust, and tolerant to high vibration and wide range of temperatures and other environmental conditions, consume little electrical energy or, equivalently, not produce excessive heat, and, on top of everything, be safe and certifiable. Consider novel airframes that are electrically propelled with emerging CONOPS, such as urban air mobility, and the constraints for low SWaP and high performance become even more important yet difficult to achieve.
Two more challenging circumstances are added when they are looking to incorporate AI into their systems. First, machine learning (ML) and neural network applications are computationally hungry beyond anything the embedded industry has seen before. And second, no ML application has yet been certified by aviation regulators – and this raises a high bar for developers on both software and hardware levels.
As we bring certifiable situational intelligence to aerospace, we are closing the gap between artificial intelligence, namely machine learning, and embedded aerospace computing. We’ve learned quite a bit along this journey.
Embedded computing professionals still need to gain experience with artificial intelligence (AI). They need to gain knowledge on this topic and may need help building systems that support and incorporate AI.
After years of working with the algorithms for the application layer and testing them on various hardware in the lab and onboard flying aircraft, Daedalean came to understand how the application capabilities can be enabled from a silicon perspective. Based on our use cases for machine learning, particularly these vision-based situational intelligence systems, we can specify their hardware requirements and offer the reference architecture – a problem statement that this market had yet to solve.
Today, Intel® published the whitepaper written in collaboration with Daedalean. We provide real-world examples of how our AI-powered situational awareness technology can be used in certifiable applications, such as vision-based traffic detection, navigation, and landing guidance included in our Situational Intelligence™ suite. Based on these use cases, we outline the specific hardware requirements sufficient to support the named capabilities.
Aircraft embedded developers, including avionics OEMs, COTS solutions providers, and airframers/systems integrators, developing computing systems face specific challenges related to airworthiness – their equipment needs to be small in size and weight, physically robust, and tolerant to high vibration and wide range of temperatures and other environmental conditions, consume little electrical energy or, equivalently, not produce excessive heat, and, on top of everything, be safe and certifiable. Consider novel airframes that are electrically propelled with emerging CONOPS, such as urban air mobility, and the constraints for low SWaP and high performance become even more important yet difficult to achieve.
Two more challenging circumstances are added when they are looking to incorporate AI into their systems. First, machine learning (ML) and neural network applications are computationally hungry beyond anything the embedded industry has seen before. And second, no ML application has yet been certified by aviation regulators – and this raises a high bar for developers on both software and hardware levels.
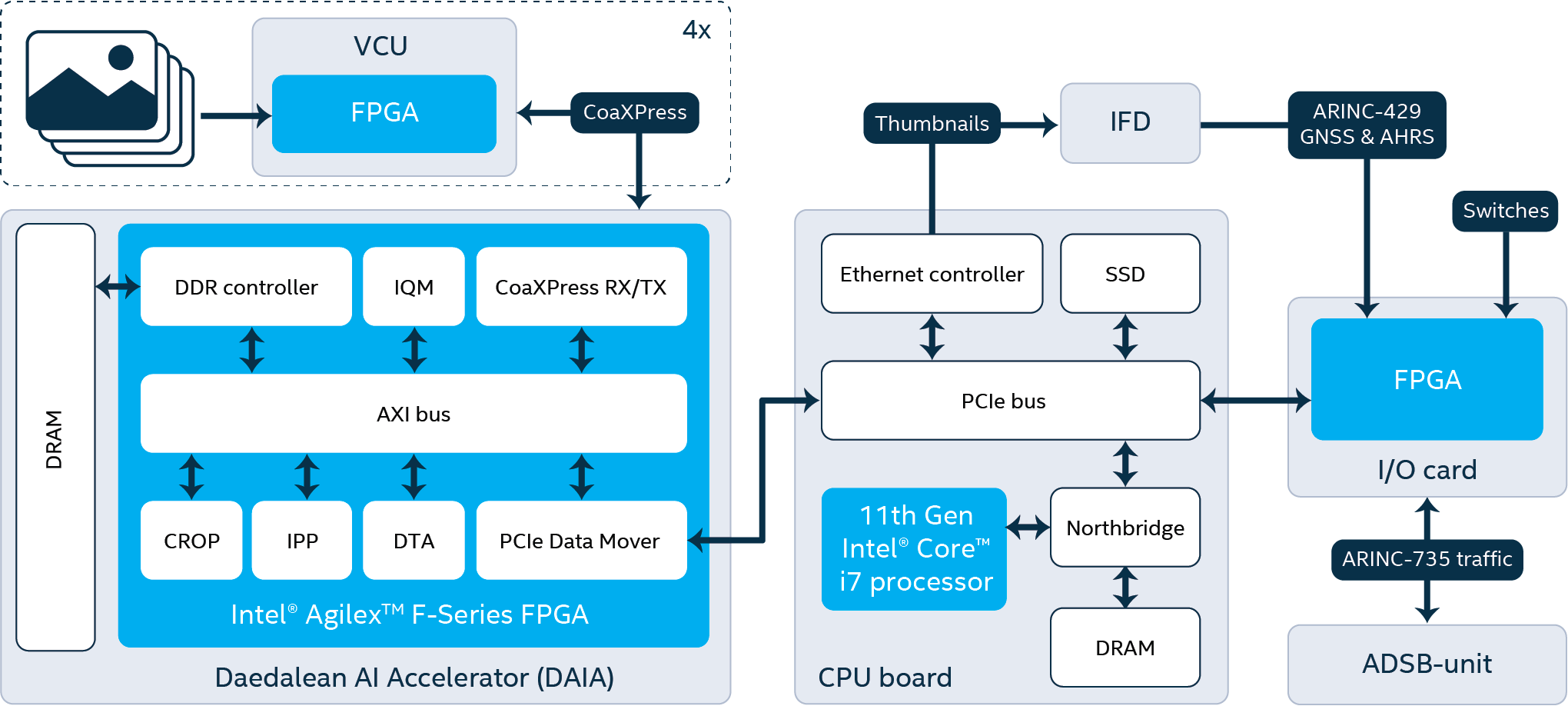
To address these challenges, we propose a reference design based on the 11th Gen Intel® Core™ i7 and Intel® Agilex™ F-Series FPGA. This architecture satisfies all requirements for future certifiable machine-learned avionics systems requiring high-performance computing at low SWaP. One example application is a vision-based situational awareness system that leverages neural networks with high-resolution, high-throughput camera inputs. We discussed key challenges and constraints limiting silicon selection in the whitepaper and provided a reference architecture based on this.
Legacy systems, such as those built on the venerable PowerPC architecture and avionics-approved AMD GPUs, were barely capable of supporting processing the frames from a single camera stream by a neural network. Moreover, the solutions that may meet the computing requirements for AI/ML applications do not meet the aviation certifiability requirements for these technologies. Without understanding the behavior of multi-core processors, system developers can struggle to introduce interference and guarantee mitigation of all potential failure conditions. For example, processor manufacturers may typically withhold details about how a multi-core processor’s shared cache works (e.g., when cache lines are evicted), despite the common understanding that cache operation significantly impacts application performance.
The proposed solution can significantly reduce time-to-market for companies interested in incorporating situational intelligence in the cockpit. But the white paper will also be insightful for any companies developing solutions in the field of certifiable embedded electronics for A&D.
Intel’s 11th gen Core™ i7 processor offers a unique advantage for aerospace suppliers since Intel has introduced the Airworthiness Evidence Package (Intel® AEP). It provides aircraft-embedded manufacturers with processor artifacts and the analysis and mitigation of non-deterministic and unintended behavior. The AEP supports DO-254 certification up to design assurance level (DAL) A.
By utilizing CPU solutions from Intel, Daedalean created a system design for a sensor computer powerful enough to process AI/ML for use in aviation applications and with the available documentation to support certification.
This whitepaper addresses the challenges of implementing ML in certifiable aerospace applications. It is the first document ever to present a real-world working example and provide guidance on how to approach these challenges in general: how to ensure that your ML-based system can meet the computational requirements, certification requirements, and the size, weight, and power (SWaP) limitations at the same time. The approach described in the document paves the way for a new generation of airworthy equipment, driving the aviation industry’s need for high-performance embedded computing.
Legacy systems, such as those built on the venerable PowerPC architecture and avionics-approved AMD GPUs, were barely capable of supporting processing the frames from a single camera stream by a neural network. Moreover, the solutions that may meet the computing requirements for AI/ML applications do not meet the aviation certifiability requirements for these technologies. Without understanding the behavior of multi-core processors, system developers can struggle to introduce interference and guarantee mitigation of all potential failure conditions. For example, processor manufacturers may typically withhold details about how a multi-core processor’s shared cache works (e.g., when cache lines are evicted), despite the common understanding that cache operation significantly impacts application performance.
The proposed solution can significantly reduce time-to-market for companies interested in incorporating situational intelligence in the cockpit. But the white paper will also be insightful for any companies developing solutions in the field of certifiable embedded electronics for A&D.
Intel’s 11th gen Core™ i7 processor offers a unique advantage for aerospace suppliers since Intel has introduced the Airworthiness Evidence Package (Intel® AEP). It provides aircraft-embedded manufacturers with processor artifacts and the analysis and mitigation of non-deterministic and unintended behavior. The AEP supports DO-254 certification up to design assurance level (DAL) A.
By utilizing CPU solutions from Intel, Daedalean created a system design for a sensor computer powerful enough to process AI/ML for use in aviation applications and with the available documentation to support certification.
This whitepaper addresses the challenges of implementing ML in certifiable aerospace applications. It is the first document ever to present a real-world working example and provide guidance on how to approach these challenges in general: how to ensure that your ML-based system can meet the computational requirements, certification requirements, and the size, weight, and power (SWaP) limitations at the same time. The approach described in the document paves the way for a new generation of airworthy equipment, driving the aviation industry’s need for high-performance embedded computing.